You'll Thank Us - 10 Tips about Saxafund.org You'll want to Know
ページ情報
照会 18回
作成日: 24-01-24 08:49
본문
Introduction:
In statistics, heteroscedasticity refers to the phenomenon where the variability of a variable of interest is not constant across the range of values of another variable. This violation of the assumption of homoscedasticity can have significant implications for statistical analyses and can lead to biased estimates, incorrect inferences, and unreliable predictions. In case you loved this post and you want to receive more info regarding Saxafund.Org please visit our page. Understanding the meaning and consequences of heteroscedasticity is crucial for researchers in various fields to ensure accurate and robust statistical analysis.
Meaning of Heteroscedasticity:
Heteroscedasticity is derived from two Greek words: "hetero" meaning different and "scedasis" meaning dispersion. It indicates that the dispersion or spread of the dependent variable is not constant across the levels of an independent variable. In simpler terms, heteroscedasticity suggests that the variability in the data does not follow a consistent pattern but rather changes as the independent variable changes. This violation of the assumption of homoscedasticity is a common occurrence in many real-world datasets.
Implications of Heteroscedasticity:
When heteroscedasticity is present, several consequences arise, affecting the accuracy and reliability of statistical analyses. These implications include biased parameter estimates, incorrect standard errors, and unreliable hypothesis tests.
Biased Parameter Estimates: Heteroscedasticity affects the Ordinary Least Squares (OLS) estimation method commonly used in linear regression analysis. Under heteroscedasticity, the OLS estimators are still unbiased, meaning they are centered on the true population values. However, they are no longer efficient and suffer from increased variability. Consequently, parameter estimates become less reliable and may deviate from their true values.
Incorrect Standard Errors: Heteroscedasticity invalidates the assumption of homoscedastic errors, leading to incorrect standard errors for estimated coefficients. Standard errors are used to calculate confidence intervals and conduct hypothesis tests. In the presence of heteroscedasticity, standard errors tend to be underestimated when the variability of the errors is higher in certain regions of the independent variable. This, in turn, leads to artificially smaller p-values, potentially resulting in a higher likelihood of rejecting null hypotheses.
Unreliable Hypothesis Tests: As mentioned earlier, heteroscedasticity can distort the results of hypothesis tests. When standard errors are underestimated due to heteroscedasticity, the t-statistics used to test the significance of estimated coefficients become inflated. Consequently, variables that are actually insignificant may appear to be significant, leading to erroneous conclusions.
Addressing Heteroscedasticity:
Detecting and addressing heteroscedasticity is vital to ensure the validity of statistical analyses. Several diagnostic tests, such as the Breusch-Pagan test and the White test, are available to detect heteroscedasticity. Once identified, there are several strategies to address heteroscedasticity, including transforming the variables, using weighted least squares estimation, or employing robust standard errors.
Conclusion:
Heteroscedasticity, the violation of the assumption of homoscedasticity, poses significant challenges in statistical analysis. Its presence can lead to biased parameter estimates, incorrect standard errors, and unreliable hypothesis tests. Researchers must be aware of this phenomenon and take appropriate steps to detect and address heteroscedasticity to ensure accurate and robust statistical analyses. By doing so, researchers can enhance the validity and reliability of their findings, leading to more meaningful scientific advancements.
In statistics, heteroscedasticity refers to the phenomenon where the variability of a variable of interest is not constant across the range of values of another variable. This violation of the assumption of homoscedasticity can have significant implications for statistical analyses and can lead to biased estimates, incorrect inferences, and unreliable predictions. In case you loved this post and you want to receive more info regarding Saxafund.Org please visit our page. Understanding the meaning and consequences of heteroscedasticity is crucial for researchers in various fields to ensure accurate and robust statistical analysis.
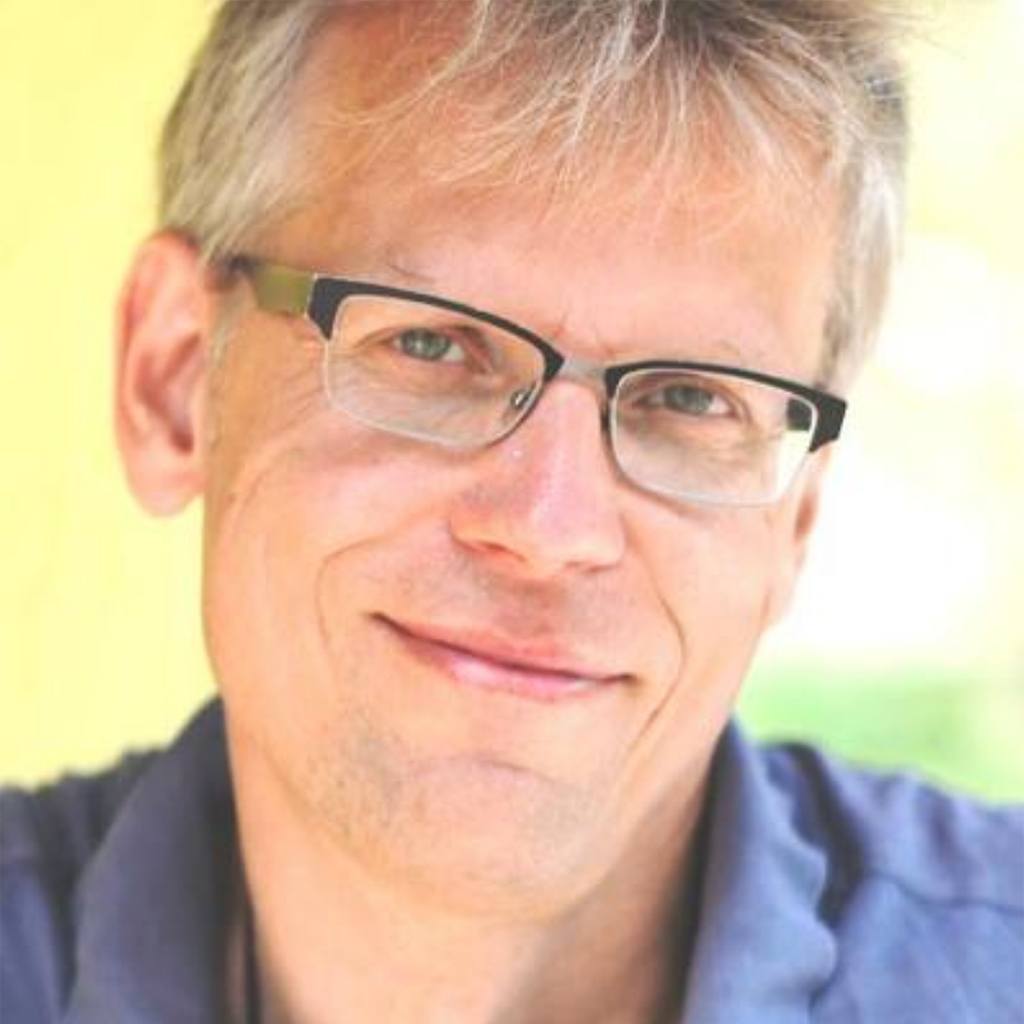
Heteroscedasticity is derived from two Greek words: "hetero" meaning different and "scedasis" meaning dispersion. It indicates that the dispersion or spread of the dependent variable is not constant across the levels of an independent variable. In simpler terms, heteroscedasticity suggests that the variability in the data does not follow a consistent pattern but rather changes as the independent variable changes. This violation of the assumption of homoscedasticity is a common occurrence in many real-world datasets.
Implications of Heteroscedasticity:
When heteroscedasticity is present, several consequences arise, affecting the accuracy and reliability of statistical analyses. These implications include biased parameter estimates, incorrect standard errors, and unreliable hypothesis tests.
Biased Parameter Estimates: Heteroscedasticity affects the Ordinary Least Squares (OLS) estimation method commonly used in linear regression analysis. Under heteroscedasticity, the OLS estimators are still unbiased, meaning they are centered on the true population values. However, they are no longer efficient and suffer from increased variability. Consequently, parameter estimates become less reliable and may deviate from their true values.
Incorrect Standard Errors: Heteroscedasticity invalidates the assumption of homoscedastic errors, leading to incorrect standard errors for estimated coefficients. Standard errors are used to calculate confidence intervals and conduct hypothesis tests. In the presence of heteroscedasticity, standard errors tend to be underestimated when the variability of the errors is higher in certain regions of the independent variable. This, in turn, leads to artificially smaller p-values, potentially resulting in a higher likelihood of rejecting null hypotheses.
Unreliable Hypothesis Tests: As mentioned earlier, heteroscedasticity can distort the results of hypothesis tests. When standard errors are underestimated due to heteroscedasticity, the t-statistics used to test the significance of estimated coefficients become inflated. Consequently, variables that are actually insignificant may appear to be significant, leading to erroneous conclusions.
Addressing Heteroscedasticity:
Detecting and addressing heteroscedasticity is vital to ensure the validity of statistical analyses. Several diagnostic tests, such as the Breusch-Pagan test and the White test, are available to detect heteroscedasticity. Once identified, there are several strategies to address heteroscedasticity, including transforming the variables, using weighted least squares estimation, or employing robust standard errors.
Conclusion:
Heteroscedasticity, the violation of the assumption of homoscedasticity, poses significant challenges in statistical analysis. Its presence can lead to biased parameter estimates, incorrect standard errors, and unreliable hypothesis tests. Researchers must be aware of this phenomenon and take appropriate steps to detect and address heteroscedasticity to ensure accurate and robust statistical analyses. By doing so, researchers can enhance the validity and reliability of their findings, leading to more meaningful scientific advancements.